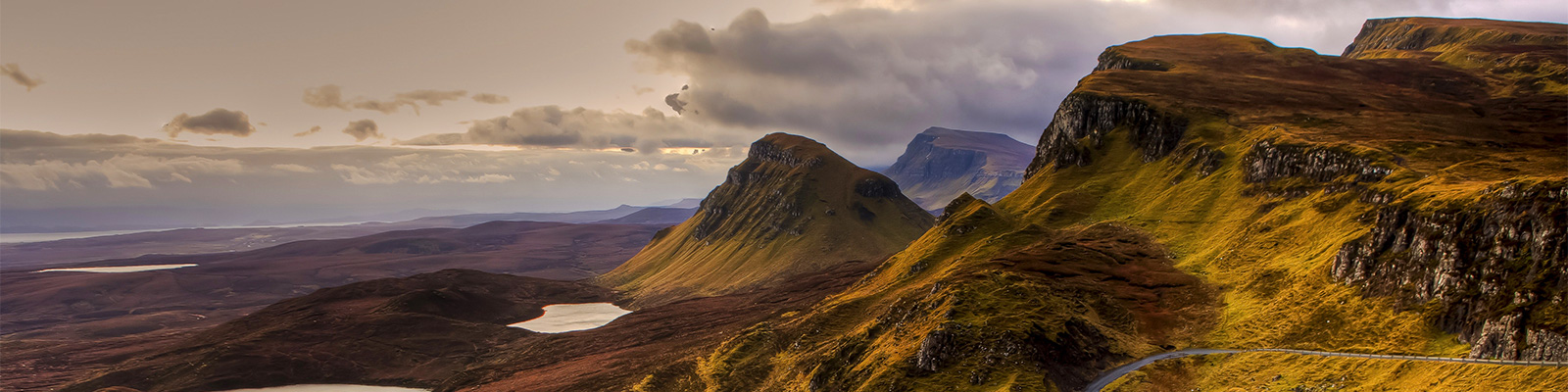
We are a group of statisticians who develop innovative statistical methodology driven by contemporary problems in the environmental sciences and ecology. Our work has led to new scientific understanding of many different physical and chemical processes and ecological systems. Such findings lead to impact in the real world. We work with data on complex processes and systems, for which off-the-shelf statistical models are overly simplistic and often computationally infeasible. Our group intersects with the Bayesian and Computational Statistics, Time Series and Changepoints, and Extreme Value Theory groups.
Environmental Modelling
Many branches of statistics, including spatial modelling, extreme value analysis and time series modelling, have their roots in Environmental Sciences applications. Our methodological and modelling innovations in these and related areas are driven by contemporary challenges in areas from space weather storms to ice sheet melt, and from air quality to coastal flooding.
Data
There has been a recent explosion in high-resolution spatio-temporal data sets measuring many aspects of our natural environment. Sources include in-situ measurements, satellite images, and mathematical model output e.g. reanalysis models, climate models, process-based forecasting models. Modern data sets include measurements made at different spatial and temporal resolutions and obtained from different sources. Traditional statistical models are not well designed for this volume of data, nor for data fusion.
Rare events
Rare, or extreme, events include river or coastal flooding, hurricanes, heatwaves, air pollution events, and space weather events. Whilst they are rare, such events have major impacts on the natural environment, society and the economy. Understanding such events is vital in local, national and global resilience planning. Unfortunately, measurements on rare events are scarce. With the extreme value group we seek to develop novel methods to describe the behaviour of such events, using spatio-temporal extreme value models. Current topics of interest include formulation of risk measures for rare events in a changing world scenario; attribution of drivers for extreme events and
Climate change
Understanding and mitigating the effects of climate change is a common thread running through many disciplines within the environmental sciences. In addition to the quantification of risk under climate change, we are interested in developing statistical methodologies to (1) identify long-term trends which may be attributable to climate change (see also time-series and change point analysis), (2) downscale output from reanalysis, regional and global climate models and (3) to provide alternative assessments for the performance climate models. The last of these points is of particular interest since most process models are designed and calibrated to reproduce average climate behaviour, potentially at the expense of other characteristics of the process, e.g. extreme events.
Complex processes
Many, if not all, environmental data sets are the product of highly complex generating mechanisms. Unlike physical process models, which are based on deterministic mathematical representations of the physical and/or chemical generating mechanisms, statistical models are based on stochastic generating mechanisms. Nevertheless, a common theme across much of our work is how to accurately represent these mechanisms. Examples of suitable frameworks include regression-type models - both parametric or semi-parametric, time-series and change point analysis, functional data analysis, and latent process models.
Ecological Modelling
At this time of biodiversity crisis, it is essential that decision-makers are provided with robust estimates to reliably inform conservation strategies, and this motivates the ecological modelling which is conducted within our group. New statistical models are being developed for a wide range of data types to ensure maximum utility of the available data.
Data
Many diverse types of data are collected on animal populations. If members of the population are individually identifiable (either through natural markings or tags/rings which can be attached to individuals) then records can be made of which individuals are captured during multiple survey occasions (capture-recapture data). Alternatively multiple sampling areas might be surveyed to assess the presence or absence of species (occupancy data); or counts of all observable members of the population (survey or census counts). More recently, higher resolution data is becoming available due to technological developments, for example satellite data, camera-trap data, biologging and GPS data.
Multi-state modelling
When capture-recapture data are collected, additional information on the location or behavioural status of an individual (e.g. breeding/non-breeding; or disease status) may also be recorded. It is possible to estimate transition probabilities between states and survival probabilities in different states through the fitting of multi-state capture-recapture models. In addition, state-uncertainty can be accommodated within the statistical model, allowing valuable insight into partially observed populations.
Translocation modelling
Conservation translocations, defined as the deliberate movement of organisms from one site to another with beneficial outcomes at population, species, or ecosystem level, are being used as a tool for the conservation of threatened species. In order to mitigate against translocation failure, it is essential that robust population size estimates of both source and post-translocation populations can be obtained. A current project in collaboration with the Mauritian Wildlife Foundation and the Zoological Society of London and the ARIES DTP aims to improve the statistical methods used on these types of data.
Integrated population modelling
Several types of data are often collected on the same population, and historically these different data types had been analysed in isolation. However, analysing data separately discards valuable information that could improve the estimation of biological quantities of interest. Integrated population modelling is a term which encompasses the simultaneous analysis of population abundance and demographic rates within a single modelling framework. There are several interesting challenges associated with integrated population modelling: accounting for variability in quality and quantity of data, accommodating different geographical and temporal scales and development of computational approaches.
Model assessment and diagnostics
Statistical models are mathematical descriptions of the data collection processes and hence are only valid if often stringent model assumptions have been met. It is essential that the validity of such assumptions are fully explored. Additionally, it is possible to construct many different models for a particular data set and it is important to fully explore the set of potential models to find the model which is best supported by the data. Failure to assess the suitability of a model might result in biased estimates which can lead to erroneous conclusions being drawn for the population of interest. Therefore, these are important steps within the model fitting process to obtain estimates which can reliably inform future conservation strategies.
-
Group Members
Loading People
Projects
Enhancements to design and testing of Body Aspect's breast volume measurement software (A4I)
01/09/2023 → 31/01/2024
Research
Quality Control Test efficiency
01/03/2023 → 30/09/2023
Research
Sub-millimetre accuracy of 3D measurements and texture analysis of Diabetic Foot Ulcers
01/03/2023 → 31/08/2023
Research
New stochastic models to quantify illegal trade
01/02/2023 → 31/01/2024
Research
Detecting soil degradation and restoration through a novel coupled sensor and machine learning framework (extension)
15/01/2023 → 14/07/2024
Research